Revenue Management: Mistakes to Avoid and Strategies to Apply
by francesco d'acunto
Distortive variables in the context of hotel statistics are factors that can influence or alter the interpretation of the collected data, leading to incorrect conclusions. Here are some practical and relevant examples in the hotel sector:
- Seasonality
- Local events
- Location
- Type of customers
- Previous online reviews
- OTA portal policies
- ‘Exogenous Shocks’ (pandemics, conflicts, global economic crises)
But the most insidious and perhaps underestimated variable is the cancellation rate.
Cancellations are not simply an operational inconvenience, but a key variable that can distort forecasts, falsify historical comparisons and compromise pricing decisions. Depending on the analytical context, cancellations can behave in two distinct ways:
1. Statistical noise
When building predictive demand models, cancellations act as noise. In other words, they generate false signals that can lead the system to overestimate real demand. In this case, new generation Revenue Management Systems (RMS) tend to:
- Exclude non-finalised bookings from the datasets
- Consider only the ‘clean’ data of bookings that have actually been finalised
This strategy allows us to generate more reliable forecasts based on actual behaviour.
2. Distorting variable
In the current on-the-books comparison vs. the same day last year, cancellations become a distorting variable. Why? Because they alter the perception of the booking pace and therefore influence the reading of the performance.
Example: if today I have 100 bookings for a future day, and last year I had 110 but then 30% were cancelled, I risk misinterpreting the current situation as negative - when in reality I could be at an advantage in terms of expected final bookings. Obviously there are also variables related to cancellations:Lead Time: (days between booking and check-in). In this case, the longer it is, the more likely it is that the booking will be cancelled.
- Cancellation policy
- Price dynamics: some people book and then cancel to rebook at a lower price.
We are often asked why, when making a comparison between the current situation and the situation at the same date last year, some metrics only consider bookings that have actually been finalised. This is certainly a relevant observation: finalised bookings incorporate future information that was not available at the date of comparison!
To understand this peculiarity we need to consider it from the perspective of the predictive model of revenue management. The primary objective of RMS software is to predict real demand. Therefore, to train a reliable predictive model, it is best to use ‘clean’ data that reflects what actually happened, not what seemed to happen but was then cancelled.
As we have already said, cancelled reservations are statistical noise: they represent intentions, not reality. If the system considers them, it risks:
- Overestimating demand.
- Increasing prices unnecessarily (because it ‘believes’ there is a lot of demand, which then evaporates).
But well-designed software doesn't completely ignore cancellations: many RMSs track cancellation rates by period/channel and some even calculate a ‘cancellation-adjusted demand forecast’.
A more sophisticated comparison consists of measuring active bookings on the same date, excluding cancellations that have already taken place. This approach reduces noise and provides a more accurate picture of the real expected demand, useful for tactical decisions.
Cancellations are not just a management nuisance, but a strategic factor. Ignoring them means working with partial, often misleading data. Treating them as noise in forecasting models and as distorting variables in comparative analyses is the first step towards revenue management that is truly based on solid data.
The real challenge today is not to eliminate cancellations, but to understand them, predict them and integrate them into decision-making processes.
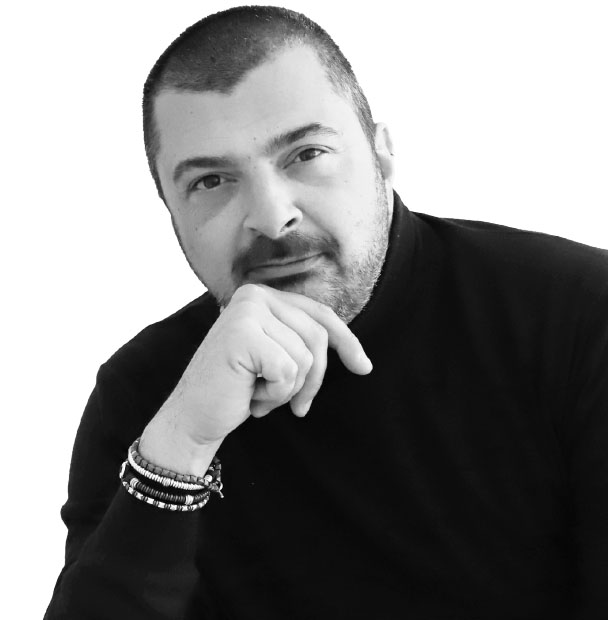